Case study 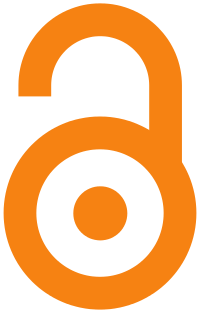
Unraveling the 2022 Sudan virus disease outbreak in Uganda: case investigations and transmission trees
Unraveling the 2022 Sudan virus disease outbreak in Uganda: case investigations and transmission trees
Richard Migisha1,&,
Paul Edward Okello1, Robert Zavuga1,
Elizabeth Katana1, Carol Nanziri1,
Lilian Bulage 1,
Rebecca Akunzirwe1,
Alex Riolexus Ario1,
Julie Rebecca Harris2
&Corresponding author
Epidemiologists play a pivotal role in outbreaks such as Ebola disease (EBOD), where understanding and interrupting transmission pathways are critical. From September to November 2022, Uganda grappled with a Sudan Virus Disease (SVD) outbreak in nine districts. The outbreak resulted in 142 confirmed and 22 probable cases. In response to this outbreak, a team of epidemiologists from the Public Health Fellowship Program were deployed to conduct case investigations to document the transmission dynamics and control the outbreak. This case study delves into the rationale behind the meticulous work of epidemiologists, emphasizing the significance of case investigations and transmission trees. As the primary architects of case definitions and data analysis, epidemiologists spearhead the effort to document a patient's course from exposure to outcome. In this context, the case study underscores the importance of transmission trees and serves as a pedagogical tool to instill in participants the vital role of epidemiologists in shaping informed decision-making during such EBOD outbreaks. This case study navigates through the complexities of building transmission trees by hand and utilizing advanced tools like the chainchecker. Participants engage in practical exercises, calculating exposure windows and constructing visual representations of transmission chains. As the outbreak unfolds, the case study guides participants in understanding the temporal aspects of disease transmission, the collaborative effort required in a multidisciplinary team, and the role of cutting-edge tools, such as chainchecker, in visualizing and confirming transmission linkages.
On September 20, 2022, the Uganda Ministry of Health declared an outbreak of Sudan virus disease (SVD) caused by Sudan virus (SUDV, species Sudan ebolavirus), after a case was identified in a 26-year-old man in Mubende District, Central Uganda [1]. Sudan virus disease (SVD) is a severe and often fatal illness affecting humans. Once the virus enters the human population-either through contact with the reservoir species (bats), or nonhuman primates or forest antelope who have been infected from bats - it can spread quickly through direct contact with body fluids from other infected persons. As of August 2022, a total of seven SVD outbreaks have been recorded globally: four in Uganda and three in South Sudan [2]. From September to November 2022, the SVD outbreak in Uganda spread from Mubende District to other districts of Uganda, including Kyegegwa, Kakumiro, Kagadi, Kassanda, Bunyangabu, Masaka, Kampala, Wakiso, and Jinja (Figure 1). By the end of the outbreak, there were 142 confirmed cases and at least 22 probable cases (persons linked epidemiologically to confirmed cases but who died without laboratory testing). On September 21, one day after the outbreak declaration, a team of fellows from the Public Health Fellowship Program (PHFP; the Advanced Field Epidemiology Training Program in Uganda) travelled to Mubende District to support the response.
Question 1: what type of expertise would you want on a case investigation team for Ebola disease (EBOD)?
The team formulated case definitions to guide case-finding and line listing, as shown below. Note: at the beginning of the outbreak, cases were only being identified in Mubende District.
Case definitions
Community case: a person with fever and no response to treatment or at least one of the following signs: bleeding (from the nose or any other part of the body, bloody diarrhea, blood in urine) or any sudden death.
Suspected case: a person with the onset of fever and no response to treatment for usual causes of fever and at least three of the following signs: headache, vomiting, diarrhea, anorexia/loss of appetite, lethargy, stomach pain, aching muscles or joints, difficulty swallowing, breathing difficulties, hiccups, or convulsions. OR illness with the onset of fever and no response to treatment for the usual causes of fever and at least one of the following signs: bloody diarrhea, bleeding from gums, bleeding into the skin, bleeding into eyes and urine, bleeding from the nose. OR any person with a history of fever (≥38°C) and at least one of the following: history of contact with a suspect, probable or confirmed SVD case, history of travel to Mubende District, or sudden/unexplained death or unexplained bleeding.
Probable case: a person who died from suspected SVD who had an epidemiological link to a confirmed case but was not tested and did not have laboratory confirmation of the disease.
Confirmed case: a suspect case with positive laboratory result for either virus antigen or to viral RNA detected by PT-PCR or IgM antibodies against Sudan virus. The team examined the case investigation forms (CIFs) that had already been completed and used them to create a basic line list. In the first week of the investigation, field teams had identified 22 confirmed and probable cases with onsets as far back as July 2022. Some of the patients had died, while others were alive and being treated in the Ebola Treatment Unit (ETU). The PHFP team was interested in linking the patients to each other to build a transmission chain. Worryingly, the team noted that some of the CIFs had no known source of infection listed for the patient.
Question 2: why is it important to quickly identify the source of infection for every case in an SVD outbreak?
Unlike some viruses, such as COVID-19 or measles, an infected person can only transmit SUDV when they are symptomatic. Before that time, a patient will not be infectious. Patients become more infectious as they become sicker and are most infectious at the time of death. When looking for the source of infection for a given SVD case, it's important to think about the sick or dead SVD patients they encountered before their illness onset. The 26-year-old male index case in Mubende District had been assigned an identifier, c008. Before c008 died, doctors suspected that he might have SVD, and had asked him about any recent contact with bats or bush meat. However, the patient had reported no such exposures. This suggested that a person infected with SUDV had been his likely exposure. But who might it have been? The CIF for c008 detailed his onset and his clinical course after admission to the hospital, but the investigation team didn't know many other details about him. The same was true for several other cases that had incomplete CIFs. To try to find the source of infection for these cases, the team knew that they needed to do some additional legwork. The first thing they did was to narrow down when each case might have become exposed. Team members knew that the incubation period of SVD was approximately 4-17 days, with a mean of approximately 6-7 days. They also knew that patients who die usually do so around 10-14 days after their illness onset. The team examined the CIFs for their 22 confirmed and probable cases and narrowed it down to a list of nine who were sick before or around the same time as c008, and who they thought could potentially be his source.
Question 3: calculate and fill in the exposure window for each case in a table.
Based on the onset dates (tabulate your answers using Table 1 as the template).
Hint: the estimated exposure window should be 4-17 days before onset. For help estimating exposure windows, we recommend utilizing the 'Ebola Exposure Window Calculator available on the App Store [3]. This user-friendly tool can assist in calculating exposure windows based on onset dates, providing a reliable reference for your case investigations. You can download it here [4].
Question 4: based on the exposure period for c008 and the onset and isolation or death dates for the other cases, which of the cases in the table is a possible source of infection for him?
Hint: the patient who serves as the source of infection for c008 has to be sick and not isolated within the time period of his exposure window (Annex 1).
Part II: case investigations and chains of transmission
The team realized that the CIFs didn't have enough information to fully understand each case. To answer their questions about who infected c008, they would need to conduct complete investigations on each case in the field, both retrospectively and moving forward. To build a fuller picture of each case, members of the case investigation team split up and went to the villages where the cases lived or had died, and health facilities where they were treated. For cases who had died, they met with family members, co-workers, and friends, as well as the healthcare staff at the facilities visited by the case. For surviving cases, they first tried to interview the case in the ETU, then went to the field to confirm what they had learned and gather additional information. The investigators wrote down every detail - they didn't know what might be useful later. The teams returned each evening to update the line list, synthesize narratives from case investigations, and attempt to represent them visually by drawing transmission diagrams (linking one case to the next). As they discussed their day's work, they realized that they were learning details in the field that were useful not only for their team but for other teams. By sharing information, they were able to make links between cases that they wouldn't have otherwise been able to make. Through their interviews in the field, they also discovered other probable cases. Many families had lost additional members but hadn't reported the deaths. After two days, they were able to gather substantial information on c008 and the other nine patients on the list above. The box on the next page has summaries of the case investigation narratives for those patients.
Summary of case narratives for 10 cases in 2022 SVD outbreak
c008: a 26-year-old male, was admitted to a private clinic (clinic F) for malaria on August 22. He was severely ill and was given intravenous antimalarial drugs, fluids, and antibiotics. After recovering, he returned home on September 4. He was readmitted to clinic F on September 11 with a 3-day history of headache, fever, vomiting, body aches, and dizziness, and was treated for presumptive malaria. On September 13, due to his worsening condition, he was referred to Mubende District Regional Referral Hospital (MRRH). He began hemorrhaging at MRRH on September 16 and was isolated and tested for viral hemorrhagic fevers. He tested positive for SVD on September 19, the day he died. Facility records showed that he shared a room with c004 at Clinic F from August 29 to September 1. Case c008 was the first confirmed case in the outbreak.
p0099: a 1-year-old female with cerebral palsy, was unwell since birth and was reportedly in and out of health facilities much of the time. Sometime in early July (her parents were not sure of exact dates, and her records were missing from the clinic), she was admitted to Clinic F in Mubende District. Around July 14, she developed fever and was admitted to Clinic F again on July 17-22 with diarrhea and vomiting. Her parents returned home with her, still unwell, on July 22, and she died at home on July 25. She is a probable case. Her infection source is unclear, but she is thought to have possibly been infected at Clinic F from another unknown patient.
p0962: a 3-year-old male, was a neighbor of p0099 and lived on the same compound. On July 21, he developed a fever, diarrhea, and abdominal pain. He was thought to have been infected through exposure to his neighbor (p0099) or possibly at clinic F. He was admitted to Clinic F from July 27 to August 2. He was referred to MRRH but went home for 3 days before going to MRRH on August 5. He died on August 8 at MRRH and was later categorized as a probable case. Hospital records showed that he was bleeding from the nose and mouth at the time of his death. He was a probable case.
c002: a 56-year-old female, had a history of hypertension and diabetes. On August 2, she was admitted to clinic F for treatment of a wound on her leg obtained during her farming activities. She was also found to be positive for malaria. On the first day of her admission (August 2), she shared a ward with p0962. She was given intravenous antibiotics, antimalarials, and fluids and discharged on August 4, having improved. On August 7, she developed a fever and headache, and on August 9 she was readmitted to Clinic F. On August 12, she was referred to MRRH, where she died on August 13. She was a probable case.
p0902: a 2-year-old male, lived in the same compound as p0099 and p0962 and was a best friend and playmate of p0962. He visited p0962 when he was home from the clinic during August 2-5. On August 11, p0902 began feeling unwell and was taken to clinic F, where he presented with vomiting and anorexia. He was admitted for 3 days and treated for presumptive malaria. He returned home but worsened, and was readmitted to Clinic F on August 18-20, and again on August 30-September 1, after which he was referred to MRRH. He died at MRRH on September 3, with bleeding from the nose and mouth. Furthermore, he was a probable case.
c001: a 6-year-old female, was at clinic F for malaria August 9-11, where she was treated as an inpatient for malaria. She was given intravenous medications, improved, and was released. On August 15, she developed fever and headache. By August 17, she was vomiting and having diarrhea. She was readmitted to Clinic F from August 17-21 and referred to MRRH, where she died on August 28. She was a daughter to c029 and a probable case.
c004: a 43-year-old female, was the sister of c002. Case c004 took care of her c002 in clinic F. She had onset on August 20 and went to Clinic F, where she died on September 1, 2022. Few additional details were available. She was a probable case.
c003: a 29-year-old female, was a neighbor of c002 and attended her funeral on August 15. On August 20, c003 began feeling ill. She developed fever, vomiting, diarrhea, stomach pain, chest pain, and joint pain, and died on September 8. The team was unable to obtain additional information about c003. She was a probable case.
c006: a 1-year-old female, was the granddaughter of c002. She attended the funeral of c002 on August 15. On August 25, she developed fever, vomiting, diarrhea, stomach pain, and rash (a common symptom of EBOD in children). She died on September 1. No additional details were available for c006.
c029: a 51-year-old male, was a small business owner. He was the caretaker to his daughter, c001, while she was ill. On August 27, he developed a headache, fever, and body aches. His illness worsened and on September 4, when he developed fever, vomiting, diarrhea, and bleeding from the nose. On September 7, he was taken to the Mulago (Kampala) Emergency Department, where he died the next day. He is likely to have contracted an infection while caring for c001.
Question 5: based on the information provided above, fill in the likeliest sources of infection for each of the cases, the transmission type (community or nosocomial), and the reason you suspect this source.
Tabulate your answers using Table 2 as the template.
Question 6: draw a transmission diagram/tree based on the above narratives. Use lines to link each case to the next.
At first, the team tried to build a transmission tree manually and transfer it to a PowerPoint slide. As new cases were identified, they shifted around the shapes and added new lines and circles. However, the constant rearrangement of a manually developed tree soon became tedious (Figure 2), and they realized they needed a new approach.
Question 7: examine the draft transmission tree above. What are the advantages of being able to visualize information this way (in a transmission chain)?
Part III - building a transmission tree
By December 1, 2022, there were a total of 176 cases of Sudan Ebola virus disease across nine districts. These cases included 142 confirmed, 33 probable, and one suspected case. Symptom onset dates ranged from July 14 to November 27, 2022. The team had been able to identify likely links for all the cases, but making the tree by hand was unwieldy. The team decided to use a software called chainchecker to build their transmission tree. Chainchecker was developed by CDC and Johns Hopkins to visualize disease transmission. It also allows the user to check the internal logic of a transmission chain by placing cases along a timeline (Does the onset and isolation date of case D fit with the exposure window date of case E? Did I list case X as a source for case Y, but case Y actually has an earlier onset date than case X?). Chainchecker also allows for visualization of health facility admissions for each case over time, which can be an important factor to assess for nosocomial transmission, as well as death dates and isolation dates [5]. The visualization allows easy identification of misplaced cases in the transmission chain and can indicate a need for greater case investigation or modification of the assigned source case. To use Chainchecker, the minimum dataset needed is a case ID and illness onset date. To have the software build a transmission chain, a 'source' field is also needed, to indicate the suspected source case for the case in question. For this file, chainchecker provides the chain below it. The dots represent onset dates for the case, and lines connect the source case to the 'recipient' case (Figure 3).
Additional information can also be added to the chainchecker diagram, such as in the figure below. The exposure window for each case (dotted purple line, health facility admission dates (thick teal and orange lines with 'H' at the start, and death dates (red skull shapes) can all be shown, as well as case classification (confirmed, probable, or suspected; not shown) and isolation dates (not shown). Chainchecker automatically calculates the exposure windows based on the onset date or, if the onset is unknown, the death date. For example, for p0099, we see an exposure window (dotted purple line) that starts in late June and ends around July 10. Chainchecker has calculated this based on her onset date, which was given as July 14, and can include it in the diagram. We can also see that p0099 stayed at a health facility July 11-12 (the short blue line before her onset dot), and July 21-22 (the short yellow line after her onset dot) before dying on July 25 (Figure 4).
Question 8: using the figure above, state the exposure windows for p0962 and p0902
Question 9: based on the data above, is p0099 a possible source for p0962? Is p0962 a possible source for p0902? Why or why not?
Question 10: besides just the exposure windows and onset dates, what other information would you need to place p0099 as a likely source for p0962?
Use the accompanying dataset in Microsoft Excel and follow the following general steps to generate transmission trees: 1) download chainchecker; 2) unzip the chainchecker zipped file; 3) open the chainchecker folder and find the file that says ’run' double-click on 'run'. It will take some time for the app to open in your browser window. If it hasn't opened after ~30 seconds, close the command window. Then try again. If you leave the command window open, it may not open in your browser window; 4) find the header that says 'data management' and click on it; 5) on the left-hand side of the window, you'll see 'upload line list' and click on it; 6) click on the paper clip shape in the opened window to select a file. Browse to find the file 'SVD_Ug_2023.xls' and select it; 7) click on the radio button for 'create new mapping'; 8) click on save mapping, in the line next to it, type ’case study mappi'; 9) map columns: a) click on the 'Epiid' dropdown and select id; b) click on ’Illness onset date’ select 'onset'; c) click on 'Epilink relationship' and select source; d) click on ’death date' and select 'death_date'; e) click on 'isolation date' and select 'date_of_isolation'; f) use the arrows to move forward one page; g) click on 'Epi case def' and select 'case_class'; h) click on 'Health care worker' and select 'hcw'; i) click on 'Hcf 1' and select 'hcf1'; j. click on 'Hcf 1 start' and select 'hcf1_start'; k. click on ’Hcf 1 End' and select 'hcf1_end'; l. do the same through Hcf 6; m. make sure 'Save mapping' is checked; n. click 'Upload'. At this point, the entire line list should appear on your screen. Click the header that reads 'chain of disease transmission'. The transmission tree should appear. Scroll up and down in the inner window to see the whole tree. You can also zoom in and zoom out to see the whole tree, or see each case individually.
Transmission tree exercises
Question 11: hover over the circle assigned to Case C001. (You may need to close the gray window first using the X on the left side). What information do you see about the case? Next, click on 'show details' in the white boxes above the tree. What happens?
Question 12: scroll to the top and locate the 'data options' section. Then select 'data layers' within this section. Click on exposure window, isolation date, and outcome date. Does the exposure window for each case make their assigned source case a true possible source? Are there any cases for which the assigned 'source case' is not able to be a source for the case that you are looking at?
Hint: for any given case, the assigned source case must have already had illness onset, not be isolated, and be alive (or newly dead, in the case of exposures to dead bodies) during the exposure window for the case of interest.
Question 13: click on 'data layers' again. Click on 'HF stay.' Hover over the yellow or teal lines to show the health facility data. Look at the health facility for the cases that occurred before September 20. What do you notice? What does this suggest to you?
On January 11, 2023, Uganda declared the end of the SVD outbreak, less than four months after the first case was confirmed in Mubende District on September 20, 2022. It was the country's first SVD outbreak in a decade and its fifth overall. In total, there were 164 officially-recognized cases (142 confirmed cases, of whom 55 died, and 22 probable, of whom all died). Overall, the case-fatality rate was 47%. Transmission trees are often built based on educated guesses, using data collected from case investigations. However, the links made can be wrong! It is always possible that another, unknown cause was in the same health facility as the case of interest and was the source of their infection, rather than the case that was known by the investigation team to be at that health facility. Or perhaps someone at home was sick and wasn't reported, and that person was the source of an infection. However, even without certainty, transmission trees play a critical role in helping investigation teams evaluate the success of their efforts, or signal where there are gaps to fill urgently.
Recent advances in whole-genome sequencing provide additional certainty about the true source of infections. Because ebolaviruses mutate over time, comparisons of the sequence of the viruses obtained between patients can provide additional information about the likelihood of one patient being the source of another's infection. Fewer genetic differences between viruses obtained from two patients indicate a higher likelihood that one was the source of the other's infection. In contrast, large numbers of genetic differences make it unlikely that one patient was the source of another's infection. In this outbreak, genetic sequencing data was available for some patients and was used to confirm and, sometimes, refute the linkages made by the investigation teams. As of 2023, thorough epidemiologic investigations along with high-quality sequencing data obtained from all patients in an EBOD outbreak is the best way to confirm transmission between patients.
The authors declare no competing interests.
All the authors have read and agreed to the final manuscript.
Table 1: cases who were ill before or around the same time as the index case (c008)
Table 2: sources of infection and transmission types for each case, along with rationale
Figure 1: map of Uganda showing the nine districts affected by Sudan virus disease during the 2022 outbreak
Figure 2: manually constructed transmission tree during the 2022 SVD outbreak in Uganda
Figure 3: A) minimum dataset needed to build a transmission chain; B) transmission diagram illustrating linkages between cases p0099, p0962, and p0902, generated using chainchecker
Figure 4: transmission diagram for cases p0099, p0962, and p0902, created using chainchecker, displaying exposure windows, health facility stays (indicated by thick teal and yellow lines with 'H' at the start), and death dates (red skull shapes)
Annex 1: template for case timelines (PDF-62KB)
- Kiggundu T, Ario AR, Kadobera D, Kwesiga B, Migisha R, Makumbi I et al. Notes from the field: outbreak of Ebola virus disease caused by Sudan ebolavirus-Uganda, August-October 2022. Morbidity and Mortality Weekly Report. 2022;71(45):1457. PubMed | Google Scholar
- Malvy D, McElroy AK, de Clerck H, Günther S, van Griensven J. Ebola virus disease. The Lancet. 2019;393(10174):936-48. PubMed | Google Scholar
- Whitesell A, Bustamante ND, Stewart M, Freeman J, Dismer AM, Alarcon W et al. Development and implementation of the Ebola Exposure Window Calculator: A tool for Ebola virus disease outbreak field investigations. Plos one. 2021;16(8):e0255631. PubMed | Google Scholar
- Miles Stewart. Ebola Exposure Window Calculator for Android. 2020. Accessed March 21, 2014.
- Gaythorpe K, Morris A, Imai N, Stewart M, Freeman J, Choi M. Chainchecker: An application to visualise and explore transmission chains for Ebola virus disease. Plos one. 2021;16(2):e0247002. PubMed | Google Scholar